Log in or create a free Rosenverse account to watch this video.
Log in Create free account100s of community videos are available to free members. Conference talks are generally available to Gold members.
Summary
Join us for a different type of Quant vs. Qual discussion: instead of discussing how data science and quantitative research methods can power UX research and design, we’re going to talk about designing enterprise data products and tools that put ML and analytics into the hands of users. Does this call for new, different, or modified approaches to UX research and design? Or do these technologies have nothing to do with how we approach design for data products? The session’s host will be Brian T. O’Neill who is also the host of the Experiencing Data podcast and founder of Designing for Analytics, an independent consultancy that helps data products leaders use design-driven innovation to deliver better ML and analytics user experiences. In this session, we’ll be sharing some rapid [slides-optional] anecdotes and stories from the attendees and then open up the conversation to everyone. We hope to get perspectives from both enterprise data teams doing “internal” data analytics or ML/AI solutions development as well as software/tech companies as well who may offer data-related platform tools, intelligence/SAAS products, BI/decision support solutions, etc. Slots are open to both experienced UX practitioners as well as data science / analytics / technical participants who may have participated in design or UX work with colleagues. Please share! If folks are too quiet in the session, you may be subject to a drum or tambourine solo from Brian. Nobody has all of this “figured out yet” and experiments and trials are welcome.
Key Insights
-
•
Designers must address the complexities of working with ML without being domain experts.
-
•
Effective communication between data scientists and designers is critical to developing user-friendly products.
-
•
Small sample sizes in ML user research introduce challenges in statistical significance.
-
•
Building trust in ML models requires transparency and interpretability.
-
•
Designers should facilitate a decision culture rather than just a data culture within organizations.
-
•
Integrated product teams can yield better outcomes when solving complex design problems in data-centric industries.
-
•
Understanding user journeys is essential for developing effective, context-aware interfaces.
-
•
Prototyping with real or realistic data can help build user trust in new products.
-
•
Designing adaptive interfaces is crucial for enhancing user interaction with predictive models.
-
•
Cross-functional collaboration allows for diverse perspectives that enrich the design process.
Notable Quotes
"If you're in user experience and you're in this space, you need to skate where the puck is going."
"Design researchers need to help turn data knowledge into insights."
"Model interpretability is often more important than accuracy, especially in business software."
"The human algorithms making decisions today can greatly inform the data models."
"We don't want to lead with a solution in search of a problem."
"Sometimes data asks for inputs that must be understood through the lens of human experience."
"Prototyping with believable data helps clarify the context for users."
"Involving designers in ML model discussions enhances trust and usability."
"Choosing the right approach is more about creating a shared space for problem-solving rather than rigid methodologies."
"Decision-making is the real need we aim to address, not just delivering analytics."
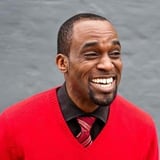
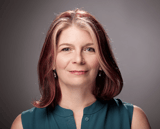
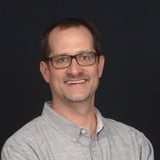
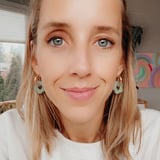
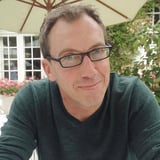
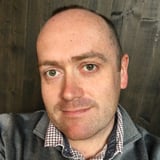
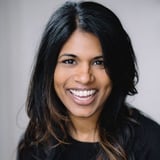
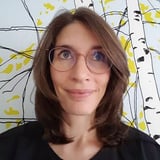
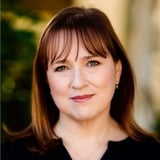
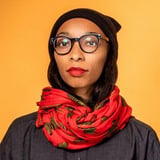
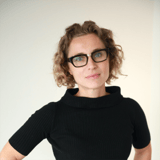
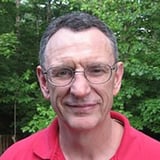
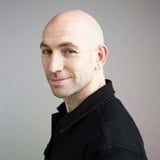

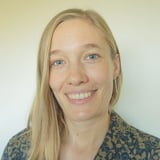
More Videos

"Friendly systems that allow for easy access and interaction can benefit all users, not just those with disabilities."
Samuel ProulxInvisible barriers: Why accessible service design can’t be an afterthought
December 3, 2024

"Live, listen, laugh, and learn while being open to transformation."
Victor UdoewaTheme One Intro
March 27, 2023

"For us to report concluded that after 33 years of research investing in design practices have a direct impact on benefits."
Gonzalo GoyanesDesign ROI: Cover a Little, Get a Lot
September 8, 2022

"Something's not right with the tech world."
Alexandra SchmidtWhy Ethics Can't Save Tech
November 18, 2022

"We have to constantly communicate what we're doing and prioritize our efforts."
Jose CoronadoFrom Zero to Hero
September 8, 2022

"Imagine the good we can do if we can reach more people."
Pippa LomasPaving the Path for Neurodiversity in Design
October 4, 2023

"Finding a community of fellow travelers can provide support and motivation in climate work."
Louis Rosenfeld Matt Jones Olga Khroustaleva Michael Leggett Karol MunozDo you want to work on climate? (Climate UX Discussion Series)
November 15, 2023

"The best organizations are the ones where design is part of the organizational value."
Jeff GothelfThe Intersection of Lean and Design
January 10, 2019

"We don't know what else is important, but we certainly expect that there will be other things that will come up as you talk about it."
Dave GrayGroup Activity: Making Sense of DesignOps
November 7, 2017