Summary
Artificial intelligence (AI) has graduated from science fiction to commoditized widget form, readily able to snap into many processes of daily life. Hence, enterprises of all maturity levels are increasingly eager to explore AI’s roles in their innovation, or outright survival strategies. Concurrently, ethical and responsible development and execution of AI-based solutions will increasingly become critical for purposes of safety and fairness. Ensuring that AI proliferates along the right path will require the infusion of multi-faceted research activities along the entire AI lifecycle. We will discuss the challenges and opportunities regarding this topic in this presentation.
Key Insights
-
•
Humanizing AI is essential for creating safe and effective AI solutions.
-
•
AI development often proceeds without comprehensive understanding, leading to real-world failures.
-
•
70% of companies report minimal impact from AI projects, highlighting significant deployment challenges.
-
•
Black box decision-making in AI can undermine trust and hinder understanding.
-
•
Bias in AI remains a significant issue, often exacerbated by limited non-technical perspectives in development teams.
-
•
AI governance frameworks need to include diverse roles, including product researchers and AI ethicists.
-
•
Visualization research can help expose intersectional biases within AI datasets.
-
•
Automated explainable AI techniques are vital for building trust in AI systems.
-
•
Multi-faceted research collaboration is necessary across academia and industry to improve AI deployment.
-
•
Proactive implementation of ML Ops can streamline AI development and enhance accountability.
Notable Quotes
"AI is not new; it's as old as computing itself, but advancements over the past 25 years have spurred its popularity."
"Most AI efforts in enterprises are failing, with nearly 90% of data science projects failing to reach production."
"AI augmentation is predicted to create $2.9 trillion in business value this year alone."
"Lack of organizational support is a significant factor in the failure of AI projects."
"Approximately 50% of AI development time is spent on data access and cleaning."
"AI bias is fueled by a lack of broad perspectives in AI solution development."
"The Gender Shades project exposed troubling disparities in facial recognition technology accuracy."
"Effective AI development requires governance, accountability, and validation processes."
"Human-centered design in AI requires integrating visualization and ethics research."
"The importance of humanizing AI will only increase as regulations around AI ethics mature."
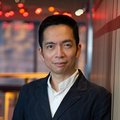
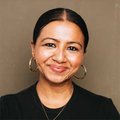
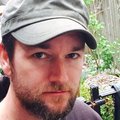
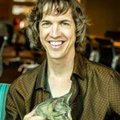
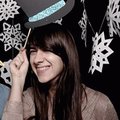
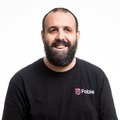
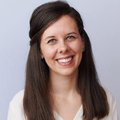
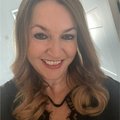
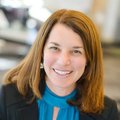
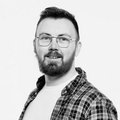
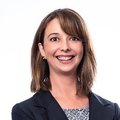
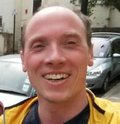
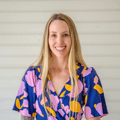
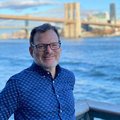
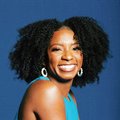
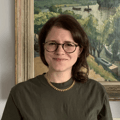
More Videos
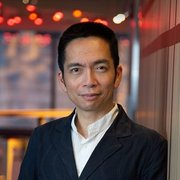
"Money is not an evil thing."
John MaedaMaking Sense of Enterprise UX
June 9, 2016
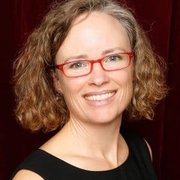
"It’s essential to approach thinking styles without prior demographic assumptions."
Indi YoungThinking styles: Mend hidden cracks in your market
January 8, 2025
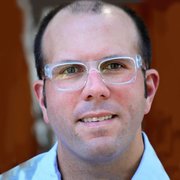
"Measuring the wrong success indicators can stifle progress and growth."
Chris ChapoData Science and Design: A Tale of Two Tribes
May 13, 2015
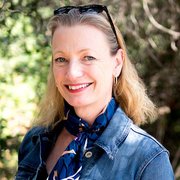
"Effective teams integrate across silos to enhance productivity."
Elizabeth ChurchillExploring Cadence: You, Your Team, and Your Enterprise
June 8, 2017
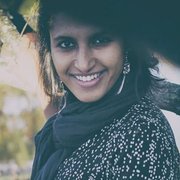
"Collectives are communities of practice individuals that have an interest in a particular topic."
Rusha SopariwalaRemote, Together: Craft and Collaboration Across Disciplines, Borders, Time Zones, and a Design Org of 170+
June 9, 2022
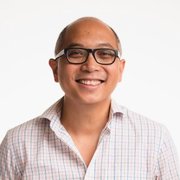
"Design helps build capital and seeks comfort."
George AyeThat Quiet Little Voice: When Design and Ethics Collide
November 16, 2022
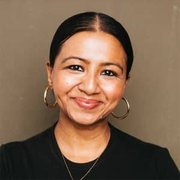
"It's our capacity to be robust under conditions of enormous stress and change that will keep us resilient."
Ariba JahanTeam Resiliency Through a Pandemic
January 8, 2024
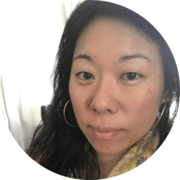
"This isn’t just about design; this is about enabling clients to succeed."
Julie Gitlin Esther RaiceDesign as an Agent of Digital Transformation at JPMC
June 9, 2021
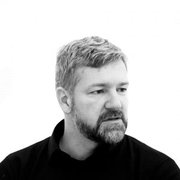
"We can decide how to improve the trust of our users based on the way we are transparent with them."
Matteo GrattonCan Data and Ethics Live Together?
October 1, 2021