Summary
AI tools like ChatGPT have exploded in popularity with good reason: they allow users to draft, summarize, and edit content with unprecedented speed. While these generic tools can generate any type of content or perform any type of content task, the user needs to craft an effective prompt to get high-quality output, and often needs to exchange multiple messages with additional guidance and requirements in order to improve results. When you’re building an AI-powered text generation feature, such as a product description or email writer, you typically can’t expect users to craft their own prompts. And unless you’re building a chat interface, you’re unlikely to offer the ability to iteratively improve the output. Instead, your feature needs a robust prompt skeleton that combines with user input to produce high-quality output in a single response. For the designer, this means building an interface that helps users provide the exact information that creates a successful prompt. This process is more complex than simple form design or a mad-lib prompt completion tool. The user input, often including free form text fields, might be required to fill in prompt variables, but it also could change the prompt structure itself, or even override base instructions. The effectiveness of the user input significantly influences the quality of the output, underscoring the need for designers to be deeply familiar with the backend prompt architecture so they can design the frontend. Drawing on recent text generation projects, I'll demonstrate how the interface design can respond to and evolve with the prompt architecture. I’ll talk about how to determine which prompt components to make invisible to the user, which to provide as predefined options, and which should be authored by the user in free-form text fields. Takeaways How prompt structure can impact user interface design and conversely, how design can impact prompt structure Techniques to provide effective user guidance within AI generation contexts to ensure consistently high-quality output Real-world examples and learnings from recent generative AI projects in an e-commerce software product
Key Insights
-
•
Designing AI text generation systems involves unique challenges compared to general chat interfaces.
-
•
Predefined prompts can lead to higher quality outputs as they guide user input and LLM behavior effectively.
-
•
The designer must balance fixed prompts with configurable user inputs.
-
•
Tone differentiation is crucial in preventing generic content across similar product descriptions.
-
•
User testing revealed that merchants struggled to articulate their brand's tone, highlighting the need for predefined options.
-
•
Names and descriptions of tones must be distinct to avoid confusion among users.
-
•
Providing rich, detailed tone descriptions improves the effectiveness of a simple dropdown selection.
-
•
Properly structuring prompts is essential for AI accuracy and relevance in text generation.
-
•
AI tools must cater to both seasoned and novice users, making design simplicity vital for new users.
-
•
Understanding the backend structure of prompts enhances user experience and output quality.
Notable Quotes
"Building interfaces for AI features is a new and exciting challenge for product designers."
"You need to know what to ask for, how to ask for it, what information to include, what you want the output to look like."
"When you're building a specific AI feature, you don't want to make your user write their own prompt."
"Providing tone differentiated suggestions is key to ensuring merchants can differentiate themselves."
"During our user testing, we found that articulating their brand voice was much harder than expected for most people."
"We had to come up with our own list of tones, as the LLM's suggestions were inconsistent."
"Tone zones need to be distinct enough for merchants to easily spot the tone that fits their brand."
"While a single tone label can be enough, providing descriptions of tone attributes leads to even better outputs."
"The user simplifies their job by selecting a single option while benefiting from a complex set of instructions."
"Thoughtful design can simplify complex AI processes for users, leading to better outputs."
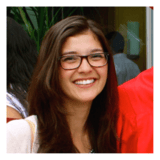
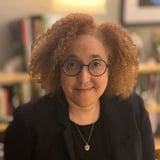
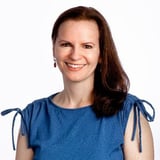
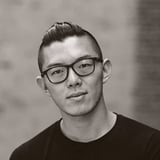
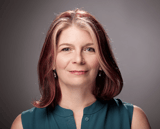
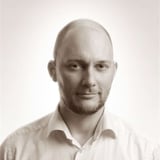
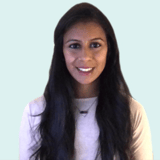
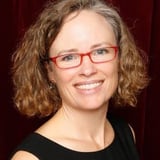

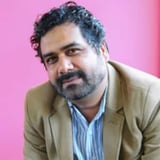
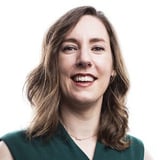
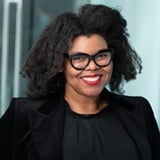
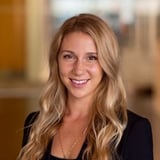
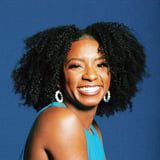
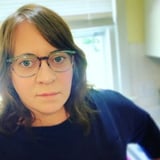
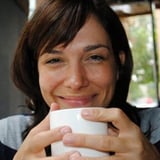
More Videos

"The misconception that accessibility is unattractive only benefits a small group is incorrect; it improves experiences for everyone."
Samuel ProulxInvisible barriers: Why accessible service design can’t be an afterthought
December 3, 2024

"I hope that today, you are transformed."
Victor UdoewaTheme One Intro
March 27, 2023

"Profit is revenues minus costs; focusing on these can help measure our contributions."
Gonzalo GoyanesDesign ROI: Cover a Little, Get a Lot
September 8, 2022

"Designers are not all-powerful; other forces shape society too."
Alexandra SchmidtWhy Ethics Can't Save Tech
November 18, 2022

"We have to constantly communicate what we're doing and prioritize our efforts."
Jose CoronadoFrom Zero to Hero
September 8, 2022

"Education will help to answer questions that people have about their own suspected neurodiversity."
Pippa LomasPaving the Path for Neurodiversity in Design
October 4, 2023

"It’s important to build enthusiasm around climate solutions to inspire action."
Louis Rosenfeld Matt Jones Olga Khroustaleva Michael Leggett Karol MunozDo you want to work on climate? (Climate UX Discussion Series)
November 15, 2023

"In a complex organization, HR is the gatekeeper to successful enterprise-wide transformation."
Jeff GothelfThe Intersection of Lean and Design
January 10, 2019

"We're all figuring it out together, making it up as we go along."
Dave GrayGroup Activity: Making Sense of DesignOps
November 7, 2017